Revolutionizing Genomic Research: The Role of AI in Genomics
Discover how AI in Genomics transforms disease diagnosis, personalized medicine, and research. Explore benefits, applications, and future trends today!

In the era of precision medicine, the integration of advanced technologies is transforming the landscape of genomic research. Among these innovations, AI in Genomics stands out as a groundbreaking tool that enhances our ability to decode the complexities of the human genome. By leveraging artificial intelligence, researchers and healthcare professionals can analyze vast amounts of genomic data with unprecedented speed and accuracy, leading to significant advancements in disease diagnosis, treatment personalization, and our overall understanding of genetic disorders. This article explores the profound impact of AI in genomics, highlighting its applications, benefits, challenges, and future prospects.
Understanding AI in Genomics
AI in Genomics refers to the application of artificial intelligence technologies, such as machine learning, deep learning, and natural language processing, to analyze and interpret genomic data. Genomics, the study of an organism's complete set of DNA, including all of its genes, generates massive datasets that are both complex and intricate. Traditional data analysis methods often fall short in handling the sheer volume and complexity of genomic information. AI, with its ability to learn from data patterns and make predictions, offers a powerful solution to these challenges.
By automating data processing and enhancing analytical capabilities, AI enables researchers to uncover hidden patterns, identify genetic variations associated with diseases, and accelerate the pace of genomic discoveries. This synergy between AI and genomics is paving the way for personalized medicine, where treatments and interventions are tailored to an individual's unique genetic makeup.
Key Applications of AI in Genomics
-
Genomic Data Analysis:
- Variant Calling and Annotation: AI algorithms improve the accuracy of identifying genetic variants (mutations) and annotating their potential impact on protein function and disease risk.
- Genome Assembly: AI enhances the reconstruction of genomes from fragmented DNA sequences, facilitating the creation of complete and accurate genomic maps.
-
Disease Diagnosis and Prediction:
- Genetic Disorder Identification: AI models can identify genetic mutations linked to specific disorders, enabling early diagnosis and intervention.
- Cancer Genomics: AI assists in classifying cancer types based on genetic profiles, predicting tumor behavior, and identifying potential therapeutic targets.
-
Personalized Medicine:
- Treatment Optimization: AI analyzes an individual's genomic data to predict responses to various treatments, allowing for the customization of therapies to maximize efficacy and minimize adverse effects.
- Pharmacogenomics: AI helps in understanding how genetic variations influence drug metabolism and efficacy, guiding the selection of the most suitable medications for patients.
-
Drug Discovery and Development:
- Target Identification: AI identifies potential genetic targets for new drugs by analyzing genomic data and understanding the underlying mechanisms of diseases.
- Biomarker Discovery: AI assists in discovering biomarkers that can predict disease progression and treatment response, accelerating the drug development process.
-
Genomic Research and Knowledge Expansion:
- Functional Genomics: AI explores the functional roles of genes and regulatory elements, enhancing our understanding of gene expression and regulation.
- Comparative Genomics: AI facilitates the comparison of genomes across different species, shedding light on evolutionary relationships and conserved genetic elements.
-
Data Integration and Interpretation:
- Multi-Omics Integration: AI integrates genomic data with other omics data (proteomics, metabolomics, etc.), providing a holistic view of biological systems and disease mechanisms.
- Clinical Decision Support: AI-powered tools offer actionable insights by correlating genomic data with clinical information, supporting healthcare providers in making informed decisions.
Benefits of AI in Genomics
-
Enhanced Accuracy and Efficiency:
- AI algorithms process and analyze genomic data with high precision, reducing the likelihood of errors and increasing the speed of data interpretation.
-
Scalability:
- AI systems can handle large-scale genomic datasets, making it feasible to analyze entire genomes and population-level data without compromising on speed or accuracy.
-
Cost Reduction:
- By automating complex data analysis tasks, AI reduces the need for extensive manual intervention, lowering the overall costs associated with genomic research and personalized medicine.
-
Accelerated Discoveries:
- AI accelerates the identification of genetic variants, disease associations, and therapeutic targets, speeding up the research and development pipeline.
-
Personalization of Healthcare:
- AI-driven genomic analysis enables the creation of personalized treatment plans, enhancing the effectiveness of therapies and improving patient outcomes.
-
Improved Data Integration:
- AI facilitates the integration of diverse data sources, providing a comprehensive understanding of genetic information in the context of broader biological and clinical data.
Challenges of Implementing AI in Genomics
-
Data Privacy and Security:
- Handling sensitive genomic data requires stringent privacy and security measures to protect against breaches and unauthorized access. Ensuring compliance with regulations like GDPR and HIPAA is crucial.
-
Data Quality and Standardization:
- The accuracy of AI models depends on the quality and consistency of the input data. Variations in data collection methods and standards can affect the reliability of AI-driven insights.
-
Technical Complexity:
- Developing and deploying AI models for genomics requires specialized expertise in both AI and genomics, posing a barrier to widespread adoption.
-
Interpretability and Transparency:
- AI models, especially deep learning algorithms, can be complex and opaque. Ensuring that the results are interpretable and transparent is essential for clinical acceptance and trust.
-
Ethical Considerations:
- The use of AI in genomics raises ethical questions regarding consent, data ownership, and the potential for genetic discrimination. Addressing these concerns is vital for responsible AI implementation.
-
Integration with Clinical Workflows:
- Seamlessly integrating AI tools with existing clinical workflows and EHR systems can be challenging, requiring careful planning and collaboration between stakeholders.
Best Practices for AI in Genomics
-
Ensure Data Privacy and Security:
- Implement robust data encryption, access controls, and anonymization techniques to protect sensitive genomic data.
-
Maintain High Data Quality:
- Standardize data collection and processing methods to ensure consistency and accuracy, enhancing the reliability of AI models.
-
Foster Collaboration:
- Encourage collaboration between AI experts, genomic researchers, and clinicians to develop models that are both technically robust and clinically relevant.
-
Invest in Training and Education:
- Provide comprehensive training programs for healthcare professionals and researchers to effectively utilize AI tools and interpret their outputs.
-
Prioritize Transparency and Interpretability:
- Develop AI models that offer clear and understandable explanations for their predictions, fostering trust and acceptance among clinicians and patients.
-
Adopt Ethical Guidelines:
- Establish and adhere to ethical guidelines that address issues of consent, data ownership, and the responsible use of AI in genomics.
-
Continuous Monitoring and Validation:
- Regularly validate AI models against new data and update them to ensure their continued accuracy and relevance in the evolving field of genomics.
Future Trends in AI in Genomics
-
Integration with Multi-Omics Data:
- Future AI models will integrate genomic data with other omics data (proteomics, metabolomics, etc.), providing a more comprehensive understanding of biological systems and disease mechanisms.
-
Advancements in AI Algorithms:
- The development of more sophisticated AI algorithms will enhance the accuracy, speed, and interpretability of genomic data analysis, enabling deeper insights and more precise predictions.
-
Personalized Genomic Medicine:
- AI will drive the evolution of personalized genomic medicine, tailoring treatments and preventive strategies to an individual’s unique genetic profile and health status.
-
Real-Time Genomic Analysis:
- Real-time genomic analysis powered by AI will enable immediate insights and interventions, particularly in critical care settings where timely decision-making is crucial.
-
AI-Driven Genomic Research Platforms:
- The creation of AI-driven research platforms will facilitate large-scale genomic studies, accelerating the discovery of genetic variants and their associations with diseases.
-
Ethical AI Frameworks:
- The development of ethical AI frameworks will guide the responsible use of AI in genomics, ensuring that advancements are made while respecting patient rights and societal values.
-
Enhanced Interoperability:
- Improved interoperability standards will enable seamless data exchange between different AI and genomic systems, fostering collaborative research and comprehensive data analysis.
Conclusion
AI in Genomics is revolutionizing the field of genetic research and healthcare by enabling the analysis of complex genomic data with unprecedented accuracy and speed. From disease diagnosis and personalized medicine to drug discovery and operational efficiency, the applications of AI in genomics are vast and transformative. Despite the challenges related to data privacy, technical complexity, and ethical considerations, the benefits of integrating AI into genomic research and clinical practice are undeniable. As technology continues to advance, the synergy between AI and genomics will drive further innovations, paving the way for a future where healthcare is more personalized, efficient, and effective.
By embracing best practices, fostering collaboration, and addressing the inherent challenges, healthcare organizations and researchers can fully harness the potential of AI in Genomics, leading to significant advancements in medical science and improved patient outcomes. The future of genomics is undoubtedly intertwined with artificial intelligence, promising a new era of discovery and precision in healthcare.
Frequently Asked Questions (FAQ)
1. What is AI in Genomics?
AI in Genomics refers to the use of artificial intelligence technologies, such as machine learning and deep learning, to analyze and interpret genomic data. This integration enhances the ability to identify genetic variants, predict disease risks, and develop personalized treatment plans.
2. How does AI improve disease diagnosis in genomics?
AI improves disease diagnosis by analyzing complex genomic data to identify patterns and mutations associated with specific diseases. This leads to earlier and more accurate diagnoses, enabling timely interventions and personalized treatment strategies.
3. What are the key benefits of using AI in Genomics?
Key benefits include enhanced diagnostic accuracy, improved patient outcomes, cost efficiency, accelerated drug discovery, personalized medicine, better data management, and informed decision-making based on comprehensive data
4. What challenges do researchers face when implementing AI in Genomics?
Challenges include ensuring data privacy and security, maintaining high data quality, integrating AI with existing genomic systems, addressing technical complexity, overcoming skill gaps, managing high implementation costs, and navigating ethical considerations related to AI and genetic data.
5. How can healthcare organizations ensure the successful implementation of AI in Genomics?
Healthcare organizations can ensure successful implementation by prioritizing data privacy and security, maintaining high data quality, fostering collaboration between AI experts and genomic researchers, investing in training and education, adopting transparent and interpretable AI models, adhering to ethical guidelines, and continuously monitoring and validating AI models.
6. What role does machine learning play in AI in Genomics?
Machine learning plays a crucial role by enabling AI systems to learn from vast amounts of genomic data, identify patterns, make predictions, and improve their accuracy over time. This enhances the ability to detect genetic variations, predict disease risks, and develop personalized treatments.
7. Can AI in Genomics be used for personalized medicine?
Yes, AI in Genomics is a cornerstone of personalized medicine. By analyzing an individual's genetic makeup, AI can help tailor treatments and preventive strategies that are specifically designed to meet the unique needs of each patient, enhancing the effectiveness and reducing adverse effects of therapies.
8. How does AI assist in drug discovery within Genomics?
AI assists in drug discovery by identifying potential drug targets through the analysis of genomic data, predicting the efficacy and safety of drug compounds, and accelerating the screening process. This leads to faster development of new medications and more efficient drug discovery pipelines.
9. What future advancements are expected in AI in Genomics?
Future advancements include the integration of multi-omics data, development of more sophisticated AI algorithms, real-time genomic analysis, personalized genomic medicine, blockchain for enhanced data security, and improved interoperability standards. These advancements will further enhance the capabilities and impact of AI in genomics.
10. How does AI in Genomics contribute to population health management?
AI in Genomics contributes to population health management by analyzing genetic data across populations to identify health trends, disease risks, and social determinants of health. This enables the development of targeted public health interventions, personalized preventive measures, and strategies to improve overall community health.
What's Your Reaction?


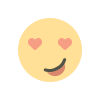


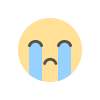
